“…drone images and vegetation index have been used as an alternative to apply only in areas where it is really necessary.”
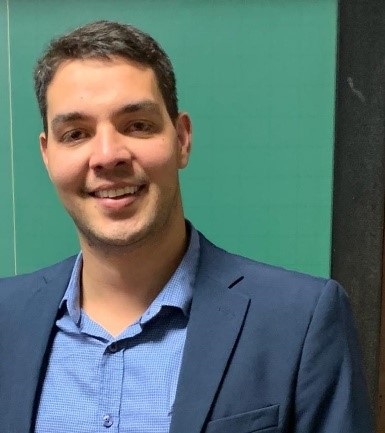
Adão Felipe dos Santos, professor at Federal University of Lavras (ESAL/UFLA).
Adão Felipe dos Santos is an agronomist from the Federal University of Tocantins, and has a master’s and doctoral degree in plant production from the Universidade Estadual Paulista Júlio de Mesquita Filho, Jaboticabal campus. Santos is a professor of precision agriculture at Federal University of Lavras (ESAL/UFLA).
The management of spatial and temporal variability (regions of a crop that presents different yield or rainfall incidence, for example) of the crops has improved the management of inputs and resources within the properties with the adoption of precision agriculture techniques. These techniques maximize the productive potential, prioritizing the allocation of resources in areas where there is a greater probability of return and, consequently, greater profitability.
Among the variability management strategies, the use of harvest maps (maps that inform the amount of product harvested in each part of the crop) from harvesters equipped with a global navigation satellite system (GNSS) can be a starting point for most farmers.
The identification of areas with greater productive potential can be easily observed through harvest maps, and together with other tools, such as satellite images, soil electrical conductivity and elevation maps, the so-called management zones (ZM) can be generated.
It is essential to use more than one crop of the crop maps, since the spatial variability of productivity may or may not be stable over time. Furthermore, for the creation of a concise and effective management zone, parameters that do not change easily, such as area relief, texture, depth of soil horizons, and water storage capacity must be considered.
With the definition of the ZM, the treatment can be done more efficiently, since it is possible to carry out applications at different rates of sowing and fertilizers, being able to recommend different genotypes and fertilization for each of the ZMs. In addition to these advantages, varied rate applications during crop development through drone images and vegetation indices have been used as an alternative to apply only in areas where it is really necessary.
An example of this type of management is the application of variable rate growth regulators to cotton. In addition, nitrogen (N) coverage applications in wheat and corn have used on-the-go sensors, which capture the reflectance of plants, generating NDVI, NDRE, GNDVI indices, and relating these values to the nutritional status of the plants for determine, through algorithms, the need to apply N in coverage.
However, in this type of prescription, zones of low productive potential will receive more fertilizer, which may not be as efficient, as it is an area of low vigor compared to the zone of high vigor. Thus, when ZM are considered in stand management, they should be associated with optimal N rate values, maximizing prescription efficiency.
Despite the advantages of using this type of technique, one of the main problems is related to the ability to analyze data and create management zones. Thus, it is essential that more professionals linked to agronomy understand the processes and know how to obtain and filter data to be used within each field.
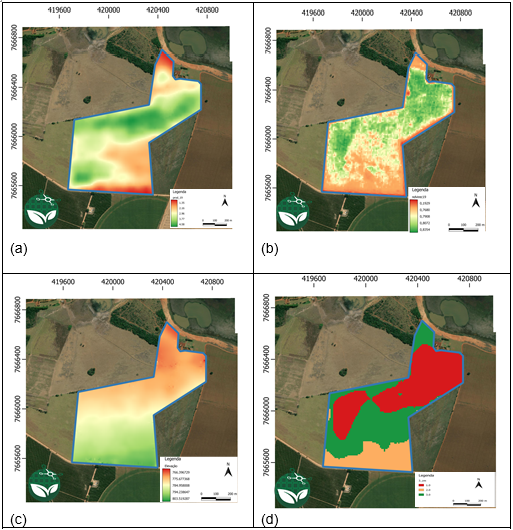
Productivity map interpolated using Krigagem after error filtering process (a) NDVI map calculated from sentinel-2 images (b), Elevation map interpolated through kriging (c); Example of definition of Management Zones considering 3 classes. **Production area in the south of Minas Gerais assisted by the Research and Extension Group in Digital Agriculture at UFLA (GEPAD/UFLA)
READ MORE: